Fraud, Transaction Monitoring 5 min read
Demystifying deepfake fraud: How the price of technology determines the targets
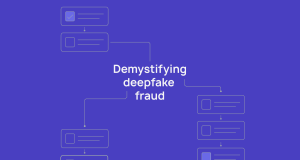
Machine learning continues to revolutionize the way fintechs and banks operate, especially when it comes to anti-fraud. While the growth of AI shows promise in effective risk decision-making, since the release of OpenAI's ChatGPT, bad actors are finding new ways to cheat the system.
"Scamming, it's going to be the growth industry of all time," said none other than investor Warren Buffet recently at his annual shareholder meeting after seeing a deepfake video of himself. The legendary billionaire added that the genie is out of the bottle, changing our world forever.
Due to the increasing prevalence of deepfake fraud, anxiety is rising among individuals and businesses - and many decision-makers in fraud prevention believe that AI is now a major contributor to identity theft.
As a risk operator, it has now become crucial to understand how and why an enterprising cybercriminal might decide to commit fraud using deepfake technology. This article will uncover the various types of deepfake fraud and the economics behind them.
The economics of deepfake fraud
Most fraudsters are rational economic actors. Each fraudulent activity can be thought of as a venture where the payoff must make up for the costs, time, and energy spent executing the scheme. This is why certain activities are more common than others: They are cheaper to pull off or more easily to scale, such as account takeovers with the help of credential stuffing.
To better understand and prepare for AI-powered bad actors, banks and fintech companies should familiarize themselves with:
1. The associated costs it takes to pull off an attack,
2. The possible attack surfaces where a given attack can be scaled,
3. The feasibility of the plan and the expected payout.
Below, we dive deep into the operational costs of various types of deepfake fraud, as this can heavily influence the different techniques that layer upon one another to perform sophisticated fraud activities.
The operational costs of deepfake fraud
Costs are determined first and foremost by the specific AI technique used, which falls into three broad categories: texts, voices, and images.
1. Text generation fraud
Text generation fraud is the most common use case for AI, commonly deployed as either chatbots or mass text generation for marketing or sales purposes.
Text generation is incredibly cheap. OpenAI prices its ChatGPT 3.5 Turbo at around $1.5 for 1M tokens of output, which is about 750,000 words. To train the language model on a similar-sized corpus, a fraudster only has to pay $0.50.
The malicious use case here is in phishing and other forms of text-based scams, where the AI can aid a cybercriminal in crafting compelling, personalized messages or simply bridge the language gap for a third-world criminal targeting first-world consumers.
In a business context, fraudsters often use text generation to impersonate an existing customer, commit invoice fraud, or Business Email Compromise (BEC) attacks.
Right now, specialized AI tools are sold on the dark web to commit all three.
A GPT model trained on fraud is priced at $200 per month and helps cyber criminals not only with phishing emails but, as it is trained on malware code, essentially acts as a virtual hacker for hire.
Criminal groups have also released a suite of tools that facilitate the defrauding of businesses through BEC. The business invoice swapper relies on the attacker having access to compromised emails, which the AI then scans for opportunities to swap invoices, with a hefty price of $2000 per week. They also offer phishing kits for tailored attacks on a company’s customers, available for a mere $999.
While text generation is cheap enough that any fraudster who knows how to bypass the guardrails can use them to “upskill” their fraud game, the specialized tools are sizeable investments for professional criminal organizations.
As such, their targets have to be big: both in terms of complex companies where they can get away with invoice fraud, or in the case of phishing campaigns they need a big enough customer list to justify the investment.
2. Voice generation fraud
Voice generation fraud occurs when a fraudster uses AI to copy someone’s voice.
The better the quality and volume of the samples used to train the system, the more believable the outcome. As such, the price range varies from $20 per month for a subscription up to hundreds or thousands of dollars for a perfect clone, like OpenAI’s Sky Voice.
In the context of financial service providers, fraudsters are using this technology to facilitate account takeovers, gain further info on their targets, or clone the customer service voice for further attacks.
Voice generation appears to be a sweet spot for scams. Not only is it relatively cheap, but companies have little opportunity to genuinely verify who they are talking to during a phone call. As a result, this type of fraud is seeing mass adoption both in consumer targeting scams and against individuals working at companies, as it is generally quite easy to scale.
3. Image generation fraud
Image generation fraud is what the public refers to as proper deepfake: Using AI to generate an individual's likeliness either as a static picture or, more commonly, in a video.
Similar to voice cloning, while simple image clones cost next to nothing, high-quality fakes range from $200 to $20,000 per minute.
As such, we have seen a rapid proliferation of scams using deepfakes, where high-profile figures have been used in scam ads to lure victims into either giving up their personal details or wire money to criminals.
Naturally, the onboarding process in financial services becomes particularly vulnerable to fraudulent actors using image generation fraud. Deepfakes have made the synthetic identities significantly harder to detect.
When it comes to KYC checks during onboarding, deepfakes tend to take two forms: presentation and injection.
Presentation is relatively low-tech, as it involves using a physical printed picture or a second screen to present the deepfake to the system to beat it, while injection refers to manipulating the in-software stream itself.
The speed of adoption of these attacks must be counter-weighed against tried and true old-school methods, like stealing documents or forging them for the same effect. A US ID with a selfie, for example, costs just $110, making it competitive with the more high-tech solutions on the market. Whereas extraordinary investments like multi-minute-long deepfake videos require an extraordinary payout.
Safeguarding against AI-powered fraud
It's evident that AI is becoming an integral part of the cybercriminal arsenal, primarily driven by economic incentives. In essence, fraudsters will always opt for tools that offer maximum efficiency at minimal cost.
However, companies are now more capable than ever in combating AI-driven fraud. This is not just due to their budgets for advanced technologies but also because of the procedures developed over decades of combating cybercrime.
Staying ahead of AI-powered fraud requires robust processes for determining, identifying, screening, and onboarding new customers or businesses. Since there is no one-size-fits-all solution to doing so, proactive fraud fighters are increasingly turning to advanced fraud and risk management platforms. These platforms, such as Taktile's next-generation risk decision platform, allow for rapid experimentation and adaptation of automated fraud detection and prevention policies - ultimately helping teams identify fraudsters better and faster.